Maximising Asset Efficiency through Machine Data Utilisation
July 27, 2023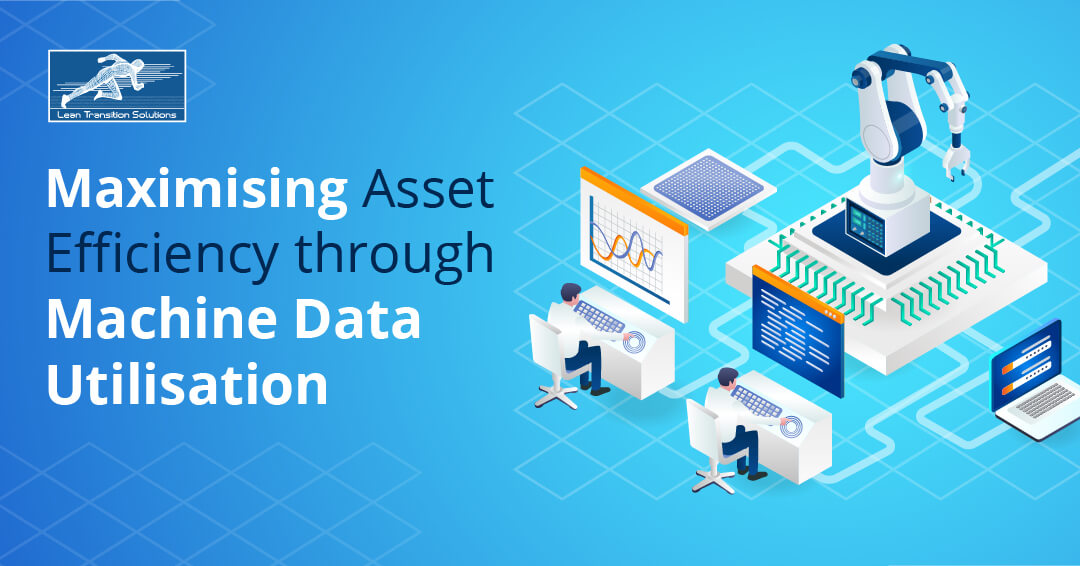
Modern businesses are in a highly competitive landscape due to globalisation, advancements in technology, ease of market access, and changing consumer preferences.Therefore, optimising asset efficiency is crucial for organisations to remain competitive. The key to achieving this goal is to tap into the potential of machine data, which is information generated by data sources within the organisation's infrastructure. By utilising this information, companies can improve their asset performance and maintenance plans, resulting in higher efficiency and cost savings.
In this blog post, we will examine how organisations can enhance their asset efficiency by utilising machine data and its benefits, like optimising asset performance, detecting issues before they become costly downtimes, and improving visibility into the condition of their assets.
What is Machine Data?
Machine data refers to the vast and diverse information generated by sensors, IoT devices, logs, and various other sources within an organisation's infrastructure. It encompasses structured, unstructured, and time-series data, providing real-time insights for proactive decision-making, predictive maintenance, and asset optimisation.
Leveraging machine data empowers businesses to streamline processes, increase productivity, enhance workplace safety, and boost employee morale. By extracting value from machine data, organisations can gain a competitive edge, driving efficiency, cost-effectiveness, and sustained growth in today's dynamic business landscape.
Types of Machine Data
- Structured Data: Structured Data is highly organised and follows a predefined format, making it easily searchable and analysable. Structured data is typically stored in relational databases and consists of rows and columns, allowing for straightforward data processing and querying.
- Unstructured Data: Unstructured machine data lacks a predefined structure, making it more challenging to analyse using traditional methods. This data includes text documents, images, videos, social media posts, and other forms of content. Although unstructured data is less organised, it contains valuable insights that can be obtained through advanced analytics and Natural Language Processing (NLP) techniques.
- Time-Series Data: Time-series data is a specific type of structured data that records information at regular intervals over time. It includes metrics such as temperature, pressure, sensor readings, and other data points collected over time. Time-series data is essential for monitoring trends, identifying patterns, and making predictions based on historical data patterns.
Common sources of Machine Data
Machine data is generated from various sources within an organisation's infrastructure.
- Internet of Things (IoT) Devices: IoT devices are interconnected smart devices, equipped with sensors that collect and transmit data over the internet. These devices, ranging from smart sensors to wearables, generate valuable data on environmental conditions, user behaviour, and operational performance.
- Sensors and Telemetry Systems: Sensors are deployed throughout industrial environments to monitor physical parameters like temperature, pressure, humidity, and motion. Telemetry systems enable remote data collection and transmission from these sensors, providing real-time insights into asset performance and conditions.
- Industrial Control Systems: Industrial Control systems (ICS) manage and automate industrial processes. They generate machine data related to process control, production rates, and operational statuses, allowing organisations to optimise manufacturing and operational efficiency.
- Operational Technology (OT) Platforms: OT platforms encompass various technologies used in industrial and operational settings, such as SCADA (Supervisory Control and Data Acquisition) systems and PLCs (Programmable Logic Controllers). These platforms generate critical machine data for monitoring and controlling industrial processes.
The role of Machine Data in Asset Management
Machine data plays a pivotal role in asset management, revolutionising traditional practices and driving businesses towards optimal efficiency and performance
1.Real-time Asset Monitoring and Condition-based Maintenance
- Predictive Maintenance using Machine Data: By analysing machine data, organisations can predict asset failures and maintenance needs before they occur, enabling proactive and cost-effective maintenance strategies.
- Reducing Downtime and Unplanned Failures: With real-time asset monitoring through machine data, businesses can swiftly respond to potential issues, minimising downtime and unplanned disruptions, thereby maximising productivity.
2.Improved Asset Performance and Efficiency
- Analysing Operational Data for Optimisation: Machine data provides valuable insights into operational performance, enabling organisations to identify improvement areas and implement data-driven decision-making.
- Identifying Bottlenecks and Inefficiencies: Businesses can pinpoint bottlenecks and inefficiencies in asset processes by analysing machine data and facilitating targeted improvements to enhance overall efficiency.
3.Enhancing Asset Lifecycle Management
- Monitoring Asset Health throughout its Lifespan: Machine data allows continuous machine health monitoring, tracking performance metrics over time, and aiding in predictive maintenance, ensuring assets function optimally throughout their lifespan.
- Planning for Asset Retirement or Upgrades: By using machine data, organisations can make informed decisions about asset retirement or upgrades, optimising asset lifecycle management and minimising unnecessary costs.
Benefits of utilising Machine Data in Asset Management
Using machine data in asset management empowers organisations to make informed decisions, optimise asset performance, and minimise downtime, ultimately driving greater efficiency, reliability, and profitability.
- Real-time insights for Proactive Decision-making: Machine data empowers businesses with real-time insights into asset performance, enabling proactive decision-making. By monitoring assets in real-time, organisations can swiftly respond to anomalies, mitigate risks, and capitalise on opportunities, enhancing Overall Operational Efficiency (OEE).
- Identifying Asset Performance Trends and Patterns: Machine data analysis allows organisations to identify key trends and patterns in asset performance. This valuable information helps understand asset behaviour over time, facilitating data-driven strategies for continuous improvement and optimising asset productivity.
- Predictive Maintenance to Reduce Downtime and Costs: One of the most significant advantages of utilising machine data is the ability to implement predictive maintenance. By analysing machine data, organisations can predict asset failures and maintenance needs, allowing them to conduct maintenance activities proactively, reducing downtime and avoiding costly unplanned breakdowns.
- Improving Asset Reliability and Efficiency: With machine data insights, organisations can optimise asset reliability and efficiency. By fine-tuning asset performance based on data-driven recommendations, businesses can enhance Overall Asset Effectiveness (OAE), increasing productivity and reducing operational costs.
Tools and technologies for analysing Machine Data
- Data Visualisation and Dashboarding Tools: Data visualisation and dashboarding tools enable businesses to present machine data in a visually appealing and easy-to-understand format. These tools allow users to create interactive dashboards and graphs, facilitating real-time monitoring and quick decision-making based on visualised data.
- Predictive Maintenance Platforms: Predictive maintenance platforms like CMMS (Computerised maintenance management Systems) utilise machine learning algorithms and advanced analytics to predict asset failures and maintenance needs. These platforms analyse historical data patterns to anticipate potential issues, enabling organisations to schedule maintenance proactively, minimise downtime, and reduce maintenance costs.
- IoT Platforms for Data Integration and Processing: IoT platforms provide a seamless ecosystem for integrating and processing machine data from various IoT devices and sensors. A Data Capture Software simplifies the collection, transformation, and storage of machine data, ensuring businesses can efficiently manage vast data streams from diverse sources.
Innovative approaches to maximise Machine Data Utilisation
- Artificial Intelligence and Machine Learning Advancements: Businesses can benefit greatly from embracing AI and machine learning advancements. These technologies allow for deeper insights to be extracted from machine data. By utilising sophisticated algorithms, AI can identify complex patterns and anomalies, which can help organisations make data-driven decisions, optimise asset performance, and predict future maintenance needs with greater accuracy.
- Integration of Machine Data with Other Business Systems: Integrating machine data with existing business systems like Enterprise Resource Planning (ERP) and Customer Relationship Management (CRM) enhances data synergy across the organisation. Combining machine data with operational and customer data gives businesses a comprehensive view of asset performance, enabling more informed decision-making and streamlined processes.
- Edge Computing and Real-time Analytics: Implementing edge computing and real-time analytics brings data processing closer to the data source, reducing latency and enabling instantaneous insights. Edge computing allows organisations to analyse machine data on-site, facilitating quick responses to critical events and optimising real-time asset performance.
Overcoming potential Pitfalls in Machine Data Utilisation
- Over-reliance on Machine Data without Human Expertise: While machine data provides valuable insights, relying solely on automated analysis without human expertise can lead to misinterpretation or overlooking crucial contextual factors. Organisations must strike a balance by involving domain experts and subject matter specialists to interpret machine data accurately and make informed decisions.
- Balancing Data-Driven Decisions with Operational Knowledge: Making data-driven decisions is crucial for efficient asset management, but balancing them with operational knowledge and experience is important. Combining machine data insights with domain expertise ensures that decisions align with operational realities, facilitating effective implementation and avoiding impractical or contradictory actions.
- Addressing Scalability Issues with Increasing Data Volumes: As data volumes grow exponentially, businesses may encounter scalability challenges in processing and managing machine data. Implementing scalable data infrastructure, leveraging cloud technologies, and adopting data compression techniques can mitigate scalability issues, ensuring smooth data utilisation and analysis.
Best practices for utilising Machine Data effectively
To unlock the full potential of machine data and achieve optimal asset management, businesses should adopt these best practices.
- Establish Clear Objectives and Goals: Define specific objectives and goals for utilising machine data, aligning them with overall asset management strategies. Clear direction ensures that data-driven efforts yield targeted outcomes and meaningful results.
- Implement Robust Data Collection and Storage Infrastructure: Build a robust data collection and storage infrastructure to ensure seamless capture and retention of machine data. This lays the foundation for efficient data processing and analysis, enabling timely decision-making.
- Utilise Advanced Analytics and Machine Learning Algorithms: Leverage advanced analytics and machine learning algorithms to extract valuable insights from machine data. Organisations can uncover patterns, trends, and correlations that drive informed asset management decisions by applying sophisticated analytical techniques.
- Implementing Predictive and Prescriptive Analytics: Predictive analytics anticipates asset failures, allowing organisations to schedule maintenance proactively and minimise disruptions. While, Prescriptive analytics recommends data-driven maintenance and operational strategies, enhancing asset reliability and performance.
- Collaborate Between Operations and Maintenance Teams: Promote collaboration and communication between operations and maintenance teams. A cross-functional approach fosters a comprehensive understanding of data requirements and ensures alignment between data utilisation and operational needs.
- Ensure Data Security and Compliance with Regulations: Safeguard machine data and protect sensitive information through robust data security measures. Comply with relevant regulations and industry standards to maintain data integrity and stakeholder trust.
Examples of Machine Data utilisation in various Industrial sectors
Here are some examples of how different industries make use of machine data.
Manufacturing Industry
In manufacturing, machine data utilisation involves real-time monitoring of production line equipment using sensors and IoT devices. By analysing machine data, manufacturers can optimise production processes, predict equipment failures, and implement predictive maintenance strategies, minimising downtime and improving overall production efficiency.
Healthcare Industry
In healthcare, machine data from medical devices and patient monitoring systems are used to improve patient care. Medical professionals rely on real-time data to monitor patient vitals, detect anomalies, and provide timely interventions. Machine data analytics also enables predictive medical equipment maintenance, ensuring uninterrupted healthcare services.
These examples demonstrate how various industries leverage machine data to optimise operations, enhance efficiency, and make data-driven decisions, contributing to improved productivity and sustainable growth.
Machine data is the backbone of modern asset management, offering real-time insights, predictive maintenance capabilities, and improved asset reliability. Businesses can extract significant value from machine data to optimise asset performance and maintenance by leveraging advanced analytics and insights derived from sensors, IoT devices, and operational logs. Real-time data analysis enables proactive decision-making, identifying asset performance trends and patterns, while predictive maintenance reduces downtime and operational costs.
Integrating machine data into asset management processes ensures improved reliability, efficiency, and lifecycle management, ultimately enhancing overall asset optimisation and driving sustainable growth. Moreover, balancing data-driven decisions with operational knowledge and human expertise ensures that asset management strategies are data-informed and practically implementable. Integrating machine data with existing business systems further enhances data synergy, providing a comprehensive view of asset performance and empowering organisations to make informed, strategic decisions.